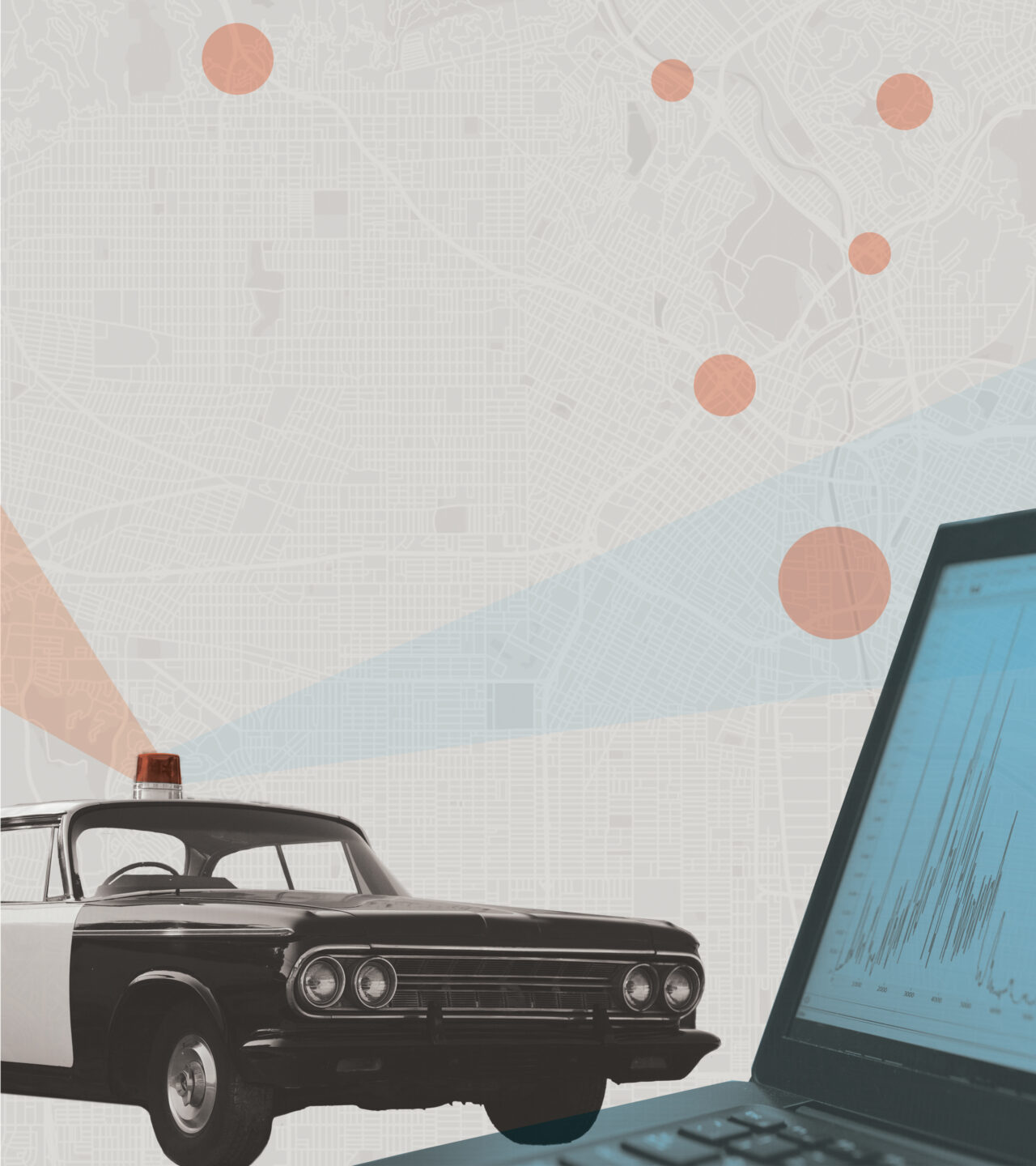
Predicting Crime
Could law enforcement be the next frontier for artificial intelligence? Professor Ryan Jenkins and a team of researchers have spent years studying the question — and how to use the technology safely.
Artificial intelligence made headlines —and generated controversy — this year when tools like DALL-E and ChatGPT rocked the art and literary worlds. But AI is also gaining ground in another area: law enforcement.
This fall, Cal Poly ethics professor Ryan Jenkins and an interdisciplinary team of researchers published a new report analyzing one emerging AI policing tool, known as place-based algorithmic patrol management — often known simply as “predictive policing.” Jenkins and his colleagues spoke with tech companies, police departments, civil rights lawyers and community leaders around the country to learn whether these systems can help solve the challenges of modern policing, and develop guidelines on how they might be used safely and effectively.
Their findings: these new systems might help — if police departments and developers act responsibly. Jenkins met with Cal Poly Magazine to explain the issues and the research team’s recommendations.
Cal Poly Magazine: What is place-based algorithmic patrol management, and what is it meant to do?
Ryan Jenkins: This is a method of using artificial intelligence to give recommendations for when and where police should patrol. ThThe idea is to forecast neighborhoods where crime is likely to take place and deploy police to the area before it happens. ThThese applications usually work by ingesting a lot of past data about the timing and the location of crimes in a city.
What are some of the challenges in policing that these systems are meant to address?
Following the death of Michael Brown in Ferguson, Missouri, and the meteoric rise of the Black Lives Matter movement in the early 2010’s, police were facing twin challenges. One was that police came under intensified scrutiny for racial bias in their behaviors — patrols, arrests, traffic stops and so on.
The other was an increased public outcry about police spending and militarization, coupled with the fact that many local governments were still reeling from the financial crash in 2008. A lot of police departments began facing unprecedented budget restrictions.
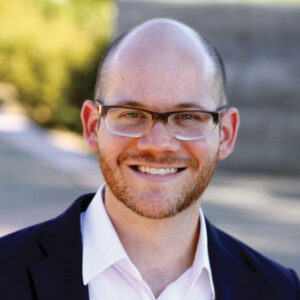
Professor Ryan Jenkins
And so along comes this technology that claims to be able to help police departments ensure that their reduced patrols are being deployed at the right place and the right time using artificial intelligence, and at the same time reduce the impact of human biases on police activity. Data-driven policing is supposed to be a solution to both of these challenges at the same time.
How effective are these systems at solving those problems?
There are lots of reasons to be optimistic. One is that data-driven policing, when it’s done right, affords an unprecedented opportunity to scrutinize the drivers of these forecasts. We can actually ask, “Why is it that police are being told to go to certain places at certain times? Does it have to do with race or not?” So, for one thing, it facilitates that kind of transparency.
Secondly, it’s able to take into account features that have nothing to do with historical crime statistics at all and hopefully eliminate any related bias from forecasts. ThThere are facts about the physical ecology of a town that can make a place attractive for opportunistic criminals. For example, liquor stores or abandoned buildings or pawn shops or bus stops or lights that are out are common predictors for crime.
If you’re using the tool, you might ask questions like, “Where are the bars and what time do they let out? Where are the large parking lots that are not lit at night?” The more we incorporate those kinds of features, the more we can dilute the problematic inheritance of historical crime data that might be biased.
What are some of the risks of this kind of technology if it is applied irresponsibly?
If it’s applied irresponsibly, it can become a form of “tech-washing” — just a nice shiny technological mask hiding the same policing practices many people have legitimate grievances about.
If that happens, you are going to end up reiterating those same biases and disproportionately burdening certain communities. Even though this technology makes it possible to reduce those kinds of biases, it still takes a very intentional effort.
Another concern is transparency. A lot of communities find this kind of technology unnerving. Quite understandably, people think of it as one step closer to Big Brother. If police-community relations are already strained, then implementing this technology might actually make things worse.
For example, the Los Angeles Police Department used a form of data-driven policing for a long time. A grassroots community movement rose up and said, “We donʼt trust this technology. We donʼt trust what the police are doing with it, and we demand an end to it.” LAPD did ultimately stop using that tool.
The police have to do a lot of additional work in terms of outreach. They need to have conversations with the community to be clear about what the technology does and doesnʼt do and how it’s being used. They have to be very forthcoming with the community about the predictions that it generates, how it generates them, and what effect it has on their patrol practices.
What are some of the major factors that your research group thinks developers and police departments need to take into consideration as they’re building or using these systems?
One important factor has to do with the data that are chosen. What kind of crimes are we targeting with the system? Some crimes — so-called “nuisance crimes” — are especially vulnerable to being infected by historical bias in police contacts and arrests. Developers can recommend, or require, that law enforcement agencies do not try to forecast crimes like those.
What kinds of algorithms are we choosing to train the crime-forecasting AI model? Some algorithms are going to be more transparent than others. If transparency is a priority for the developers or for the law enforcement departments, that’s a reason to adopt certain algorithms over others.
When youʼre thinking about how to work with police departments to create these systems, you need to think about what kind of data you’re displaying to officers and how you explain it to them. At the same time that police departments are getting skepticism from the community, they’re getting the same skepticism from their own officers, who might say things like, “I’ve been walking this beat for 20 years. I’m pretty sure I know where the crime is. I don’t need a computer to tell me where to go.”
There’s a lot of educating that needs to take place to ensure the responsible development of the system, the effective uptake and incorporation into law enforcement agencies and also to make sure that it doesn’t corrode police-community relations even further. That work needs to take place throughout the entire process of development and deployment.
What are some of the specific recommendations that your team is making to ensure responsible use of these systems?
Certain crimes, those that happen relatively frequently and are geographically correlated, are going to be easier to forecast than others. Because we have a lot of data about them, the model will be more confident about the forecasts.
But some of these crimes, which we call “nuisance crimes,” like drug possession, loitering and public intoxication, have historically been policed in a way that puts a disproportionate burden on marginalized communities and communities of color. Those are real crimes that communities might want to tamp down, but the data that we have about them might be infected with historical bias.
It’s not enough just to say, “We’ll just feed all the data we have about crimes into this thing and it’ll spit out these perfect predictions.” You have to be careful with which crimes you’re asking the algorithm to predict, and avoid feeding it data that’s likely to be infected with bias.
It’s also helpful to tell officers why they’re being asked to go to a certain area. If you’re telling them, “Go visit this area for about 15 minutes,” they might go there expecting to witness a crime. They might feel the need be on high alert. You have to think about what information officers actually need to put them at ease, and also to make it transparent to them why they’re being asked to go there.
That could include information like, “There’s been a rash of burglaries in this area. There are some abandoned buildings there, or there are pawn shops where people might be pawning stolen goods.”
Developers should also think about what they are asking officers on patrol to do. Consider, instead of looking for crimes in progress, to simply talk to community members. There might be a gate or garage door open, and the officer might go and remind residents it’s safer to keep those closed. Those are the kinds of things that are helpful and productive, and those kinds of informal conversations can also help build trust between police and community members.
It’s not about sending an officer to walk around an area with gun drawn, expecting to catch a crime in progress — it’s much more nuanced.
What are the next steps for your report, and what impact do you hope that it will make?
The next step is to get it into the hands of developers, police departments and community representatives. This report should be a guidebook to how these things work, how they can be used well or poorly, and what you should expect of law enforcement departments. For instance, one of our goals is to help community members understand what kinds of questions they should ask law enforcement agencies that want to use an application like this.
Even if those three audiences don’t adopt the whole 90-page report, at very least we hope it helps them identify some simple practices that they can use. Moving the needle even a little bit counts as real progress in an area like this.
What does moving that needle look like? Big picture, what do you hope more responsible adoption of this kind of technology will accomplish?
Police-community relations are a complicated issue, and a problem that has more than one cause needs more than one solution.
I think the gold standard is a repaired trust between police and communities. Public trust is crucial for so many facets of the criminal justice system, and if we can help to heal that trust just a little bit, it will generate safer, healthier communities. That’s something that all of us want.